분자의 합성 반응 경로를 설계하는 것은 유기 합성의 기본 측면으로, 생물 의학, 제약, 재료 산업 등 다양한 분야에 중요한 의미를 갖습니다. 역합성 분석은 합성 경로를 개발하는 데 가장 널리 사용되는 접근 방식입니다.
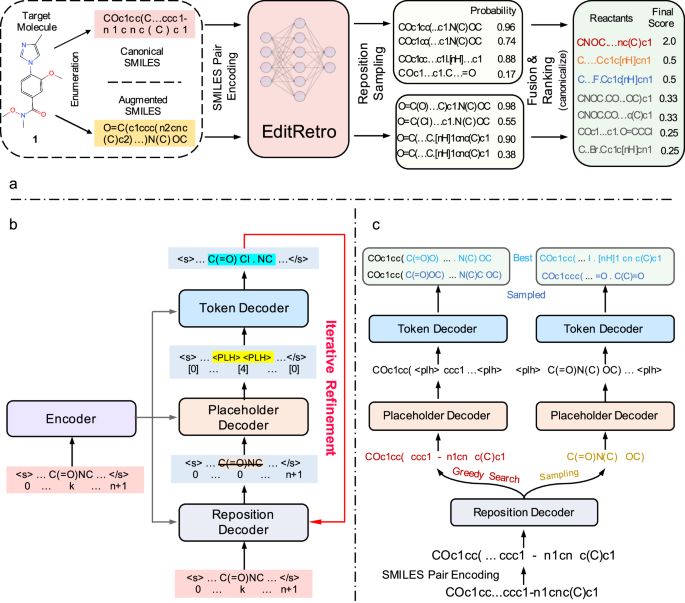
Organic synthesis plays a pivotal role in various fields, including biomedical, pharmaceutical, and materials industries. Retrosynthetic analysis serves as the primary approach for designing synthetic routes, aiming to decompose molecules into simpler precursors using established reactions. This methodology, initially formalized by Corey, led to the development of computer-aided synthesis planning (CASP). In recent years, artificial intelligence (AI)-driven retrosynthesis has facilitated the exploration of more complex molecules and significantly reduced the time and energy required to design synthetic experiments. Single-step retrosynthesis prediction is a crucial component of retrosynthetic planning, and several deep learning-based methods have been proposed with promising results. These methods can be broadly categorized into three groups: template-based, template-free, and semi-template-based methods.
유기합성은 생물의학, 제약, 소재산업 등 다양한 분야에서 중추적인 역할을 담당하고 있습니다. 역합성 분석은 확립된 반응을 사용하여 분자를 더 간단한 전구체로 분해하는 것을 목표로 하는 합성 경로를 설계하기 위한 주요 접근 방식입니다. Corey가 처음에 공식화한 이 방법론은 CASP(컴퓨터 지원 종합 계획)의 개발로 이어졌습니다. 최근 몇 년 동안 인공 지능(AI) 기반 역합성은 더 복잡한 분자의 탐색을 촉진하고 합성 실험을 설계하는 데 필요한 시간과 에너지를 크게 줄였습니다. 단일 단계 역합성 예측은 역합성 계획의 중요한 구성 요소이며, 몇 가지 딥 러닝 기반 방법이 제안되어 유망한 결과를 얻었습니다. 이러한 방법은 크게 템플릿 기반, 템플릿 프리, 반템플릿 기반 방법의 세 그룹으로 분류할 수 있습니다.
Template-based methods regard retrosynthesis prediction as a template retrieval problem and compare the target molecule with precomputed templates. These templates capture the essential features of the reaction center in specific types of chemical reactions. They can be generated manually or automatically and serve as a guide for the model to identify the most suitable chemical transformation for a given molecule. Various works have proposed different approaches to prioritize candidate templates. RetroSim employed the molecular fingerprint similarity between the given product and the molecules present in the corpus to rank the candidate templates. NeuralSym was the pioneering work to utilize deep neural networks for template selection by learning a multi-class classifier. GLN built a conditional graph logic network to learn the conditional joint probability of templates and reactants. LocalRetro conducted an evaluation of the suitability of local atom/bond templates at all predicted reaction centers for a target molecule and incorporated the non-local effects in chemical reactions through global reactivity attention. It has demonstrated state-of-the-art performance within the template-based methods. Although providing interpretability and molecule validity, template-based models suffer from limited generalization and scalability issues, which can hinder their practical utility.
템플릿 기반 방법은 역합성 예측을 템플릿 검색 문제로 간주하고 표적 분자를 미리 계산된 템플릿과 비교합니다. 이러한 템플릿은 특정 유형의 화학 반응에서 반응 센터의 필수 기능을 포착합니다. 이는 수동 또는 자동으로 생성될 수 있으며 모델이 특정 분자에 가장 적합한 화학적 변환을 식별하는 가이드 역할을 합니다. 다양한 연구에서는 후보 템플릿의 우선순위를 정하기 위한 다양한 접근 방식을 제안했습니다. RetroSim은 주어진 제품과 코퍼스에 존재하는 분자 사이의 분자 지문 유사성을 사용하여 후보 템플릿의 순위를 매겼습니다. NeuralSym은 다중 클래스 분류기를 학습하여 템플릿 선택을 위해 심층 신경망을 활용하는 선구적인 작업입니다. GLN은 템플릿과 반응물의 조건부 결합 확률을 학습하기 위해 조건부 그래프 논리 네트워크를 구축했습니다. LocalRetro는 표적 분자에 대해 예측된 모든 반응 중심에서 국소 원자/결합 템플릿의 적합성을 평가하고 전역 반응성 관심을 통해 화학 반응에 비국소 효과를 통합했습니다. 템플릿 기반 방법 내에서 최첨단 성능을 보여주었습니다. 해석 가능성과 분자 타당성을 제공하지만 템플릿 기반 모델은 제한된 일반화 및 확장성 문제로 인해 실제 유용성을 저해할 수 있습니다.
Template-free methods utilize deep generative models to generate reactant molecules without relying on predefined templates. Most of existing methods reformulate the task as a sequence-to-sequence problem, employing the sequence representation of molecules, specifically the simplified molecular-input line-entry system (SMILES). Liu et al. first utilized a long short-term memory (LSTM)-based sequence-to-sequence (Seq2Seq) model to convert the SMILES representation of a product to the SMILES of the reactants. Karpov et al. further proposed a Transformer-based Seq2Seq method for retrosynthesis. SCROP integrated a grammar corrector into the Transformer architecture, aiming to resolve the prevalent problem of grammatical invalidity in seq2seq methods. R-SMILES established a closely aligned one-to-one mapping between the SMILES representations of the products and the reactants to enhance the efficiency of synthesis prediction in Transformer-based methods. PMSR devised three tailored pre-training tasks for retrosynthesis, encompassing auto-regression, molecule recovery, and contrastive reaction classification, thereby enhancing the performance of retrosynthesis and achieving state-of-the-art accuracy within template-free methods. Some studies characterize the task as a graph-to-sequence problem, employing the molecular graph as input. Graph2SMILES integrated a sequential graph encoder with a Transformer decoder to preserve the permutation invariance of SMILES. Retroformer introduced a local attention head in the Transformer encoder to augment its reasoning capability for reactions. Recent studies, including MEGAN, MARS, and Graph2Edits, have explored the utilization of end-to-end molecular graph editing models to represent a chemical reaction as a series of graph edits, drawing inspiration from the arrow pushing formalism. However, these approaches usually require time-consuming predictions for sequential graph edit operations. Fang et al. developed a substructure-level decoding method by automatically extracting commonly preserved portions of product molecules. However, the extraction of substructures is fully data-driven, and its coverage depends on the reaction dataset. Furthermore, incorrect substructures can lead to erroneous predictions. While template-free methods are fully data-driven, they raise concerns regarding the interpretability, chemical validity, and diversity of the generated molecules.
템플릿이 필요 없는 방법은 사전 정의된 템플릿에 의존하지 않고 심층 생성 모델을 활용하여 반응물 분자를 생성합니다. 대부분의 기존 방법은 분자의 시퀀스 표현, 특히 단순화된 분자 입력 라인 입력 시스템(SMILES)을 사용하여 작업을 시퀀스 간 문제로 재구성합니다. Liu et al. 먼저 LSTM(Long Short-Term Memory) 기반 Seq2Seq(Sequence-to-Sequence) 모델을 활용하여 제품의 SMILES 표현을 반응물의 SMILES로 변환했습니다. Karpovet al. 역합성을 위한 Transformer 기반 Seq2Seq 방법을 추가로 제안했습니다. SCROP는 seq2seq 메서드에서 널리 퍼져 있는 문법적 무효 문제를 해결하는 것을 목표로 문법 교정기를 Transformer 아키텍처에 통합했습니다. R-SMILES는 제품의 SMILES 표현과 반응물 사이에 밀접하게 정렬된 일대일 매핑을 확립하여 Transformer 기반 방법에서 합성 예측의 효율성을 향상시켰습니다. PMSR은 자동 회귀, 분자 복구 및 대조 반응 분류를 포함하는 역합성을 위한 세 가지 맞춤형 사전 훈련 작업을 고안하여 역합성 성능을 향상시키고 템플릿 없는 방법 내에서 최첨단 정확도를 달성했습니다. 일부 연구에서는 분자 그래프를 입력으로 사용하여 작업을 그래프-시퀀스 문제로 특성화합니다. Graph2SMILES는 순차 그래프 인코더를 Transformer 디코더와 통합하여 SMILES의 순열 불변성을 보존합니다. Retroformer는 반응에 대한 추론 능력을 강화하기 위해 Transformer 인코더에 로컬 어텐션 헤드를 도입했습니다. MEGAN, MARS 및 Graph2Edits를 포함한 최근 연구에서는 화학 반응을 일련의 그래프 편집으로 표현하기 위해 엔드투엔드 분자 그래프 편집 모델의 활용을 탐구했으며, 화살표 추진 형식주의에서 영감을 얻었습니다. 그러나 이러한 접근 방식은 일반적으로 순차적 그래프 편집 작업에 대해 시간이 많이 걸리는 예측이 필요합니다. Fang et al. 제품 분자의 일반적으로 보존된 부분을 자동으로 추출하여 하위 구조 수준 디코딩 방법을 개발했습니다. 그러나 하위 구조 추출은 완전히 데이터 기반이며 해당 범위는 반응 데이터 세트에 따라 다릅니다. 또한 잘못된 하위 구조로 인해 잘못된 예측이 발생할 수 있습니다. 템플릿이 없는 방법은 완전히 데이터 기반이지만 생성된 분자의 해석 가능성, 화학적 타당성 및 다양성에 대한 우려를 제기합니다.
Semi-template-based methods leverage the benefits of the two aforementioned methods. These methods follow a two-stage procedure: first, fragmenting the target molecule into synthons by identifying reactive sites, and subsequently converting the synthons into reactants using techniques such as leaving groups selection, graph generation, or SMILES generation. RetroXpert first identified the reaction center of the target molecule to obtain synthons by employing an edge-enhanced graph attention network, followed by the generation of the corresponding reactants based on the synthons. RetroPrime introduced the mix-and-match and label-and-align strategies within a Transformer-based two-stage workflow to mitigate the challenges of insufficient diversity and chemical implausibility. G2Gs initially partitioned the target molecular graph into several synthons by identifying potential reaction centers, followed by the translation of the synthons into the complete reactant graphs using a variational graph translation framework. GraphRetro first transformed the target into synthons by
반템플릿 기반 방법은 앞서 언급한 두 가지 방법의 이점을 활용합니다. 이러한 방법은 2단계 절차를 따릅니다. 먼저 반응 부위를 식별하여 표적 분자를 신톤으로 단편화한 다음 이탈 그룹 선택, 그래프 생성 또는 SMILES 생성과 같은 기술을 사용하여 신톤을 반응물로 변환합니다. RetroXpert는 먼저 에지 강화 그래프 주의 네트워크를 사용하여 신톤을 얻기 위해 대상 분자의 반응 중심을 식별한 다음 신톤을 기반으로 해당 반응물을 생성했습니다. RetroPrime은 불충분한 다양성과 화학적 타당성 문제를 완화하기 위해 Transformer 기반 2단계 워크플로우 내에서 혼합 및 일치 및 라벨링 및 정렬 전략을 도입했습니다. G2G는 처음에 잠재적인 반응 중심을 식별하여 대상 분자 그래프를 여러 신톤으로 분할한 다음 변형 그래프 변환 프레임워크를 사용하여 신톤을 완전한 반응물 그래프로 변환했습니다. GraphRetro는 먼저 대상을 다음과 같이 신톤으로 변환했습니다.